Aug 9th, ‘24/10 min read
Observability vs. Telemetry vs. Monitoring
Observability is the continuous analysis of operational data, telemetry is the operational data that feeds into that analysis, and monitoring is like a radar for your system observing everything about your system and alerting when necessary.
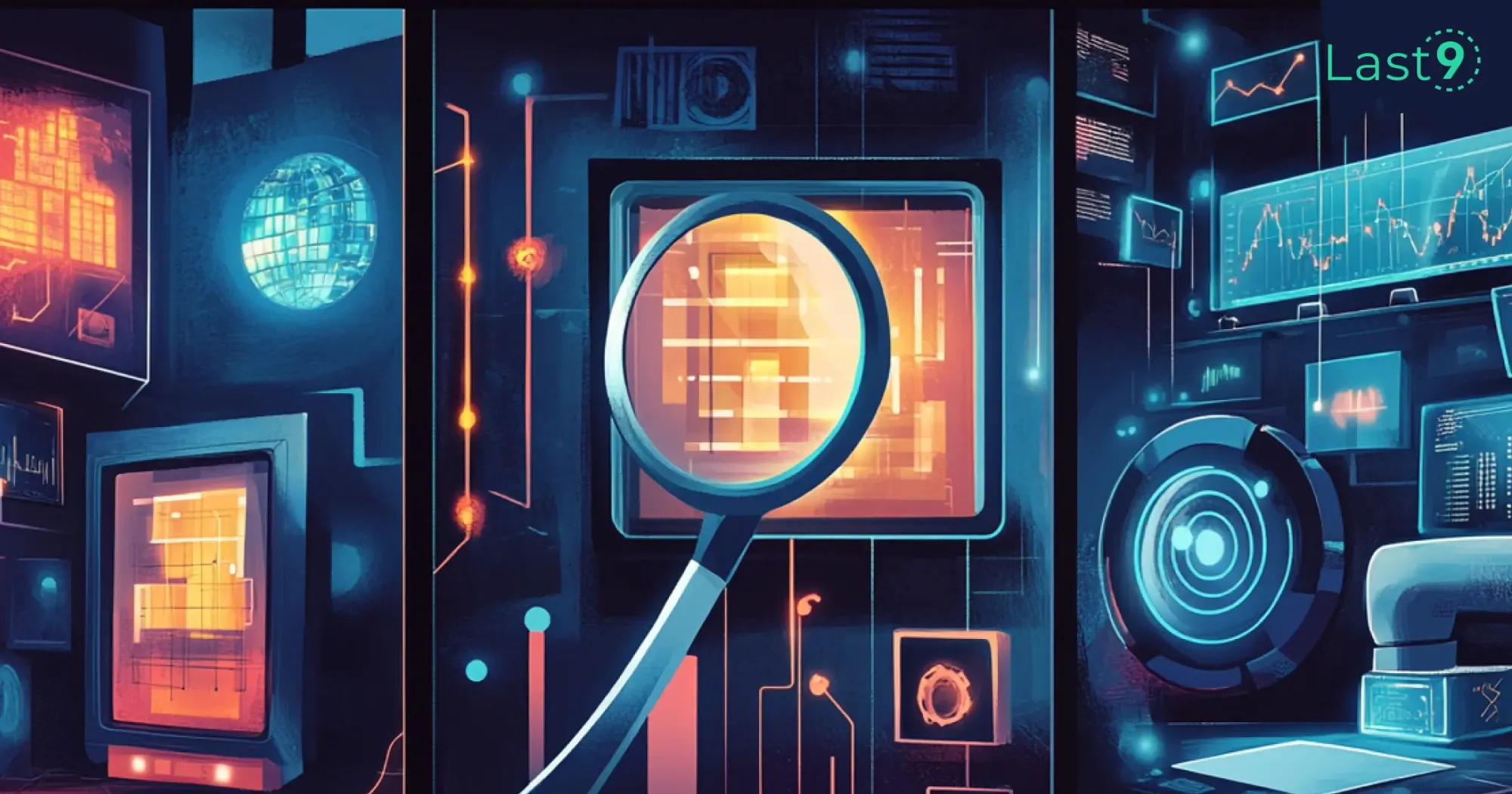
Observability, telemetry, and monitoring are crucial for efficient and reliable software development and deployment. While these terms are related, they have distinct meanings and functions.
This article explains clearly the differences between observability, telemetry, and monitoring and how they collectively contribute to system reliability.
Key Definitions
- Observability: Observability is the ability to infer a system’s internal workings from its external outputs.
- Telemetry: The automated process of collecting and transmitting data from remote sources to a central location for analysis and monitoring.
- Monitoring: The practice of continuously watching and checking the status of a system to detect problems or anomalies based on predefined metrics.
Observability is the continuous analysis of operational data, telemetry is the operational data that feeds into that analysis, and monitoring is like a radar for your system observing everything about your system and alerting when necessary.
What is Telemetry?
Telemetry is an automated process of remotely collecting and transmitting data using sensors and communication systems.
It focuses on data collection, so it does not provide analysis or insights but depends on monitoring tools to analyze collected data. Telemetry gathers various metrics—such as performance statistics, error rates, and resource utilization—to gain insights into system behavior.
Types of Telemetry
Two main types of telemetry and the data types they collect are discussed below.
Application Telemetry
This type involves adding code or instrumentation to an application to gather and transmit data about its behavior. OpenTelemetry is a commonly adopted application telemetry tool.
In OpenTelemetry, the SDK is used for initialization, while an API is used for code instrumentation. Instrumentation allows the OTel tool to gather performance, event, and resource utilization-related metrics, pooled using SDKs, and transport to application performance monitoring(APM) tools for analysis.
Here are some examples of data collected through application telemetry.
Application Telemetry Data | Description |
---|---|
Performance Metrics | Provide insights into system performance, such as response times, latency, throughput or error rates. Help assess the efficiency of and bottlenecks within the system. |
Resource Utilization Metrics | Allows for monitoring of systems' resource utilization, such as CPU usage, memory consumption or network bandwidth, to ensure resource allocation efficiency and optimization. |
Event Tracking Metrics | Tracks specific system events, including user actions and API calls. |
Network Telemetry
Network telemetry helps to monitor and optimize network behavior by collecting data related to network conditions and performance, such as bandwidth usage, speed, and other network KPIs.
Network telemetry can be collected actively (by routinely stimulating the system) or passively (by recording system events and collecting runtime data).
Some examples of network telemetry include:
Network Telemetry Data | Description |
---|---|
Traffic Volume | Measures network traffic flowing through different network components. Network telemetry helps prevent security threats by monitoring and analyzing network load. This data can be collected via software probes, network taps or other network monitoring tools/techniques. |
Latency | Captures time taken for data or requests to travel across networks. Network telemetry involves checking for signs of software latency such as recurrent delays or inconsistent spikes in application response times. Key network performance indexes in this category include jitter, packet delay variation (PDV) and round trip time (RTT). |
Packet Loss | Identifies issues that may impact data integrity or system performance by monitoring the rate at which network packets are lost during transmission. KPIs related to packet loss include packet loss rate, burst packet loss, out-of-order packets and retransmission rates. |
What is Observability?
Observability is the ability to understand and infer the internal state of a system based on its external behavior. This external behavior includes the kinds of external outputs that the data systems generate and the latency of data generation.
It involves collecting and analyzing data from various sources, including metrics, logs, and traces, to gain insights into system behavior and performance.
Observability platforms provide a comprehensive view of multi-cloud software deployment environments and cloud-native applications that help DevOps teams perform swift root cause analysis and correlation in a way that goes beyond simple monitoring.
It also provides teams with inputs so that they can establish automation pipelines. This allows for effective and proactive troubleshooting, optimization, and problem-solving, covering all use cases.
Types of Telemetry in Observability
The following are the types of telemetry commonly used in observability tools.
Metrics
Metrics provide quantitative measurements of system performance and behavior. They include data points such as response times, error rates, CPU usage, memory utilization, and network throughput.
Metrics-driven telemetry provides values that help IT teams recognize and resolve abnormal system performance for improved application health and performance.
Logs
Logs capture specific events or activities within a system. They provide detailed information about system behavior, capturing error messages, user actions, application events, and other information.
Logs also provide contextual information about metrics. With Log-based telemetry, SRE and DevOps teams can see the exact time an event occurred, why it occurred, and which other events are connected to it, making it helpful in troubleshooting and investigating performance issues.
Traces
Traces follow the flow of requests or transactions through different system components. They can track how the application or network components work together or flow across networks to deliver the desired results.
These telemetry data provide detailed insights into the execution path and timing of distributed application requests. Traces enable you to understand dependencies, analyze performance bottlenecks, and identify issues that may span multiple components.
What is Monitoring?
Monitoring is actively observing and measuring an IT infrastructure system's health and performance to ensure it functions as expected.
Monitoring involves setting up predefined checks and alerts to detect potential failures and deviations from expected behavior. However, it usually does not provide the same depth and flexibility as observability.
Prometheus is a widely regarded open-source tool as the de-facto monitoring tool in the industry, known for its robust data collection, querying, and alerting capabilities.
Types of Monitoring
In any monitoring exercise, different types of monitoring can be used individually or in combination based on specific requirements and goals. Below are some common types of monitoring.
Performance Monitoring
Performance monitoring tracks, measures, and analyzes critical application performance metrics. These include response times, error and request rates, throughput, and resource utilization (CPU and memory) metrics.
Performance monitoring thus helps to ensure optimal system performance by identifying potential bottlenecks.
Infrastructure monitoring
Infrastructure monitoring is pivotal for Site Reliability Engineering (SRE) and operations teams, ensuring systems run efficiently and reliably. It provides real-time data, enabling SREs to identify and address potential system bottlenecks or failures promptly.
With these insights, they can uphold their commitments to service level objectives (SLOs) and maintain a balance between innovation and stability. This proactive approach helps SRE teams maintain system resilience, ensuring seamless user experiences. This becomes even more critical in the case of serverless or Kubernetes-based applications where infrastructure is ephemeral.
Availability Monitoring
The most critical software monitoring metric, availability or uptime monitoring, focuses on determining if a system or its components are available and responsive.
It involves monitoring factors such as uptime, network connectivity, response status codes, and overall availability of critical functionalities. Availability monitoring can be done using a cloud HTTP or a ping sensor. It helps to detect and address system availability and connectivity issues promptly.
Log Monitoring
Log monitoring involves continuously analyzing system logs (as recorded) to identify patterns, anomalies, and potential issues. Logs provide detailed records of system events and errors, allowing monitoring tools to detect and alert on abnormal behavior.
Real-Time Monitoring
Real-time monitoring involves continuous and immediate data analysis in its current state when a command is issued or a request is made. It detects and responds to events or issues in real real-time. It involves real-time analytics and alerting systems to capture and analyze real-time data.
Security Monitoring
Security monitoring detects and mitigates potential security threats and vulnerabilities in real-time. It involves monitoring system logs, network traffic, and user behavior to identify anomalies, unauthorized access attempts, or suspicious activities with in-application runtime security.
Capacity Monitoring
Capacity monitoring involves tracking and analyzing resource usage over time to allocate system resources optimally. It includes monitoring metrics such as CPU, memory, disk space, and network bandwidth utilization.
Capacity monitoring also provides insights into resource usage variation over time by measuring data processing speed, latency, and volume. It helps DevOps teams to identify resource constraints, improve capacity planning, and optimize system performance.
Levitate is managed time series data warehouse capable of infrastructure monitoring, application monitoring, availability monitoring, capacitty monitoring, making it a compelling monitoring solution. See it in action!
Observability vs. Monitoring vs. Telemetry
Aspect | Observability | Telemetry | Monitoring |
Focus | System behavior understanding | Data collection and transmission | System status checking |
Data Type | Logs, metrics, traces | Raw data from remote sources | Predefined metrics and alerts |
Scope | Entire system state | Data collection process | Specific system aspects |
Purpose | Troubleshooting and optimization | Data gathering for analysis | Detecting known issues |
Timeframe | Real-time and historical | Real-time data collection | Real-time status updates |
While each concept is essential, it is important to note that none individually guarantees system reliability. They complement each other, and a comprehensive approach should include all three.
Telemetry provides real-time data, monitoring helps detect specific issues, and observability facilitates analysis of collected data to find root causes, understand system behavior, and optimize performance.
How They Work Together
Observability, telemetry, and monitoring are complementary practices that work together to ensure system reliability and performance:
- Telemetry collects and transmits raw data from various system components.
- Monitoring uses this data to track predefined metrics and alert on known issues.
- Observability leverages the same data to provide deeper insights and troubleshoot complex, unforeseen problems.
Telemetry vs Observability
Telemetry focuses on collecting and transmitting data from various systems in multi-cloud environments for further analysis. In contrast, observability is about understanding a system's internal state and behavior based on the available data.
It involves collecting, analyzing, and interpreting telemetry data to troubleshoot and explore complex systems. While telemetry tools are used for versatile data collection and measurement, observability provides deeper insights into the root causes of issues and guides their resolution.
Telemetry vs Monitoring
Monitoring relies on telemetry data to function effectively, while telemetry alone doesn't provide complete insights into application performance.
Telemetry collects real-time data on performance, usage, errors, and KPIs, which monitoring tools then use to track and alert system health and performance.
Unlike telemetry, which provides comprehensive metrics across multi-cloud environments, traditional monitoring tools depend on predefined metrics set by developers.
Observability vs Monitoring
Observability offers a detailed view of system events, enabling DevOps teams to trace data from user requests to system responses for precise root cause analysis and remediation.
In contrast, monitoring provides a broad view of system health by continuously collecting and aggregating data on predefined metrics, focusing on specific performance aspects.
How to Choose the Right Observability and Monitoring Tools
Choosing the right tools for telemetry depends on several factors, including your specific requirements, the nature of the system or application being monitored, and the goals of your telemetry implementation.
Here are some checks to guide you in selecting the appropriate tools:
Criteria | Description |
Telemetry Requirements | Identify the types of data you need to collect, such as metrics, logs, events, and traces. Define your goals, like performance optimization, error detection, or capacity planning. |
System Architecture and Data Sources | Understand your system’s architecture and data sources. Consider storage capacity, costs, and options for long-term archiving. Ensure the tool supports efficient querying and retrieval. |
Compatibility and Integration | Check if the telemetry tools work with your existing infrastructure, languages, frameworks, and databases. Ensure they integrate with your application stack and third-party observability solutions. |
Scalability and Performance | Ensure the tool can handle large volumes of data from various sources. Look for features like load balancing and clustering for large-scale deployments. |
Ease of Use and Flexibility | The tool should be easy to set up and manage. It should also allow for custom metrics and functionality through APIs or plugins. |
Analytics and Visualization Capabilities | Evaluate the tool’s ability to analyze and visualize data. Look for flexible querying and features like dashboards, charts, and reports. |
Security and Compliance | Ensure the tool meets security and compliance standards, including encryption and access control. |
Vendor Reputation and Future Roadmap | Research the vendor’s reputation and customer reviews. Assess their commitment to product development and future feature enhancements. |
Support and Community | Evaluate available support options, such as documentation, forums, or ticket-based systems. Consider the size and engagement of the user community. |
Conclusion
Observability, telemetry, and monitoring are essential for maintaining system reliability.
A comprehensive approach that includes design principles, fault tolerance, and proactive maintenance is crucial.
Integrating these elements enables the collection of detailed telemetry data, monitoring of application health, and in-depth root cause analysis. This approach leads to faster anomaly detection, reduced downtime, and enhanced system performance, ultimately benefiting business outcomes.
FAQs related to Observability vs. Telemetry vs. Monitoring
1: What’s the difference between telemetry and monitoring?
A: Telemetry involves collecting and transmitting data. Monitoring uses this data to track and detect issues in real time.
2: How does observability differ from monitoring?
A: Monitoring tracks specific metrics and alerts on known issues. Observability allows you to explore data and understand complex system behavior beyond predefined metrics.
3: How do telemetry, monitoring, and observability work together?
A: Telemetry collects and transmits data, monitoring tracks specific metrics and alerts on known issues, and observability uses that data to understand and troubleshoot complex problems. Together, they provide a complete picture of system health and performance.
4: What role does telemetry play in system performance optimization?
A: Telemetry provides the data needed to identify performance bottlenecks and inefficiencies. By analyzing this data, you can make informed decisions to optimize system performance and enhance overall reliability.
5: Can observability tools replace traditional monitoring tools?
A: Observability tools complement traditional monitoring tools by providing deeper insights and the ability to explore data beyond predefined metrics. They are not a replacement but an enhancement that allows for more comprehensive analysis.
6: What are some common challenges in implementing observability?
A: Common challenges include integrating with existing systems, managing large volumes of data, ensuring data quality, and creating meaningful visualizations. Addressing these challenges requires careful planning and the right tools.
7: How do AI and ML enhance telemetry and observability?
A: AI and ML can analyze large volumes of telemetry data to identify patterns, detect anomalies, and predict potential issues. This enhances the effectiveness of observability by providing more accurate and timely insights.
8: What factors should be considered when choosing telemetry tools?
A: Considerations include compatibility with your existing infrastructure, scalability, ease of use, analytics and visualization capabilities, security, and vendor reputation.
9: How can organizations ensure they are using telemetry data effectively?
A: Organizations should focus on defining clear goals for data collection, implementing robust data analysis practices, and regularly reviewing and adjusting their telemetry strategy to ensure it meets their evolving needs.
Newsletter
Stay updated on the latest from Last9.
Helping to make the tech a little less intimidating. I love breaking down complex concepts into easy-to-understand terms.
Handcrafted Related Posts
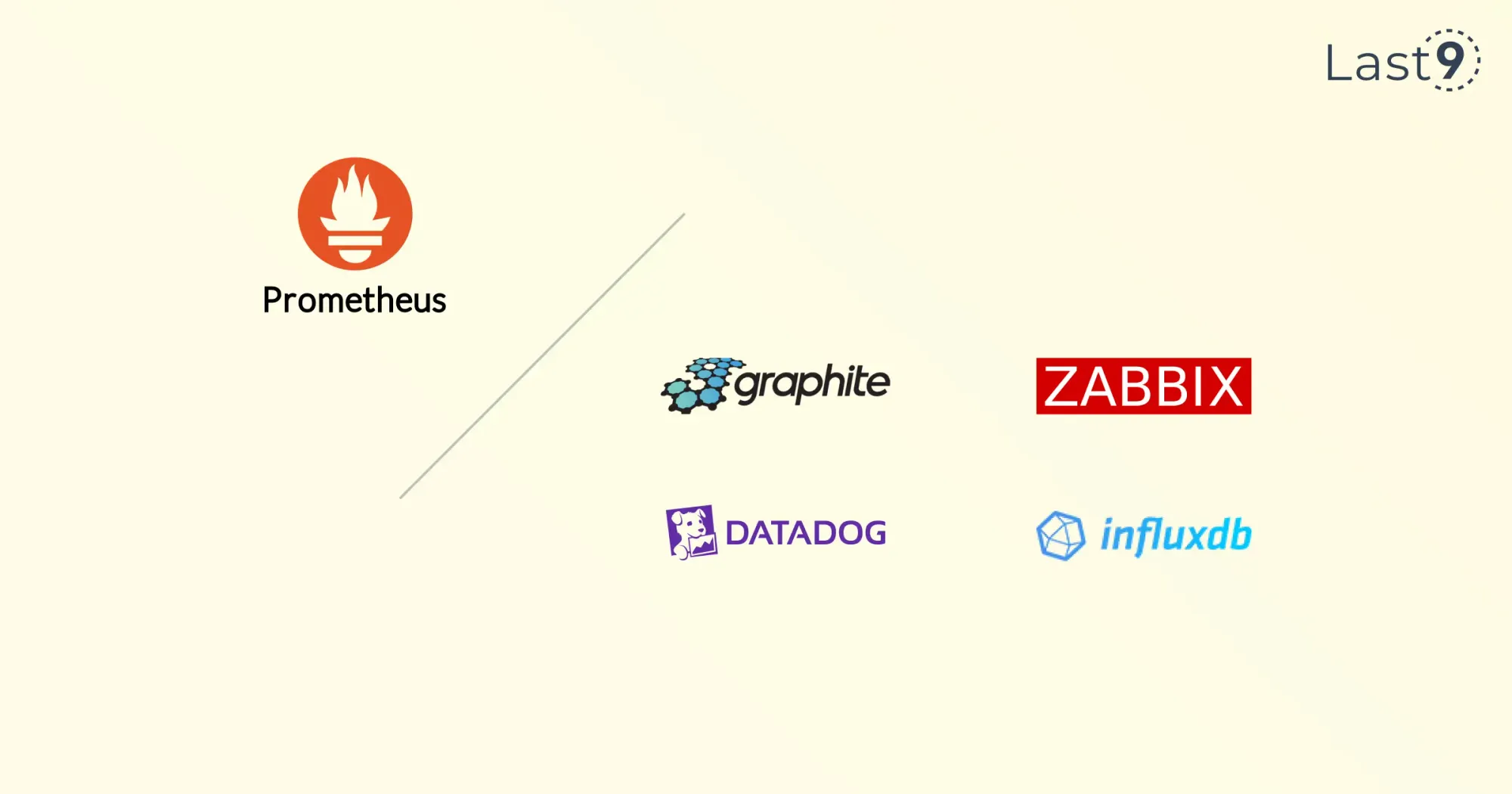
Prometheus Alternatives: Monitoring Tools You Should Know
What are the alternatives to Prometheus? A guide to comparing different Prometheus Alternatives.
Gabriel Diaz
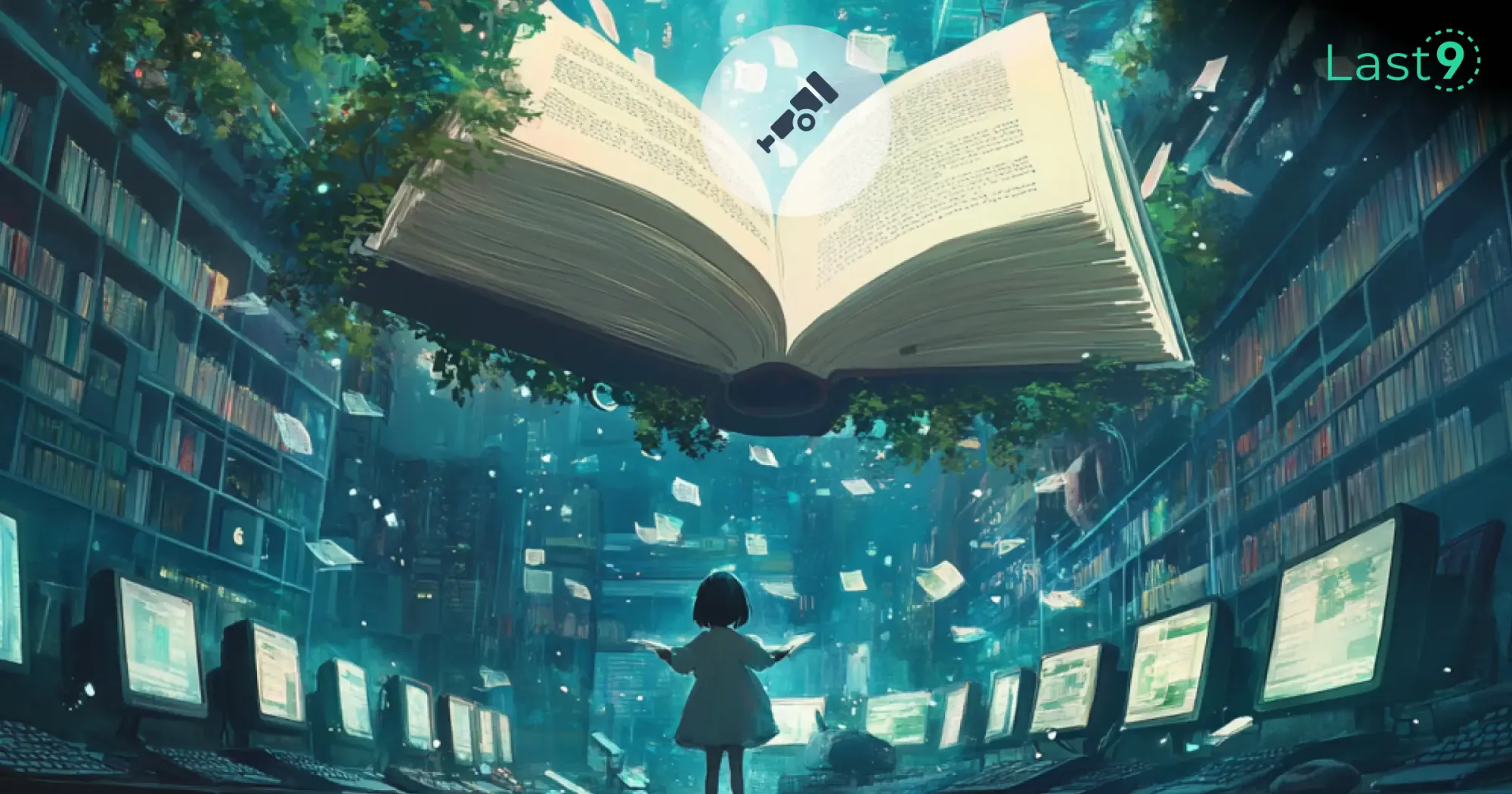
OpenTelemetry Collector: The Complete Guide
This guide covers the OpenTelemetry Collector, its features, use cases, and tips for effectively managing telemetry data.
Prathamesh Sonpatki, Anjali Udasi
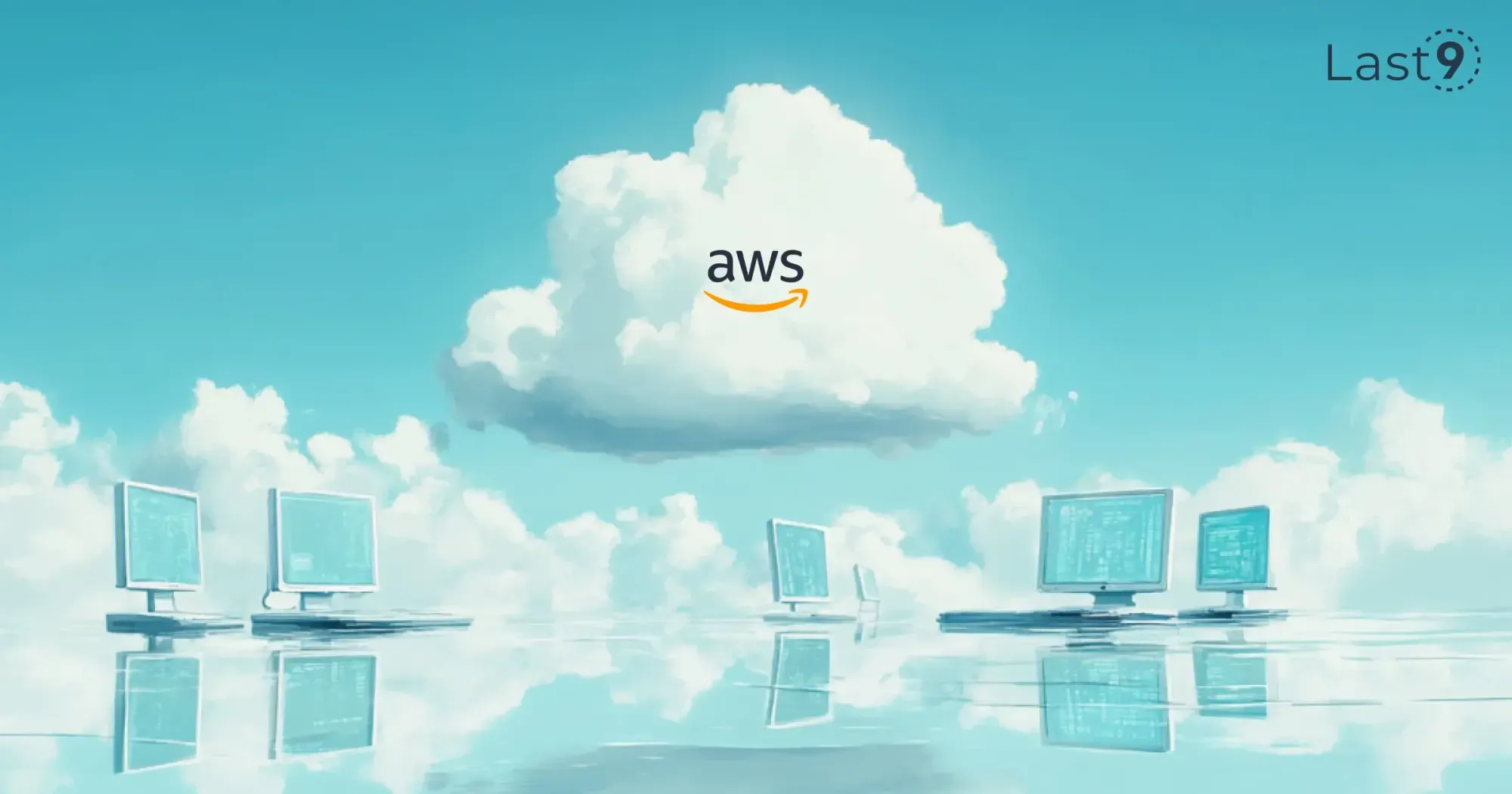
AWS CloudTrail Guide: Uses, Events, and Setup Explained
Learn how AWS CloudTrail tracks user activity, logs events, and helps with compliance. Get insights on setup and best practices.
Anjali Udasi