Jan 10th, ‘25/13 min read
Why Data Observability is Important for Your Business
Learn how data observability helps your business catch issues early, ensuring accurate insights, smarter decisions, and smoother growth.
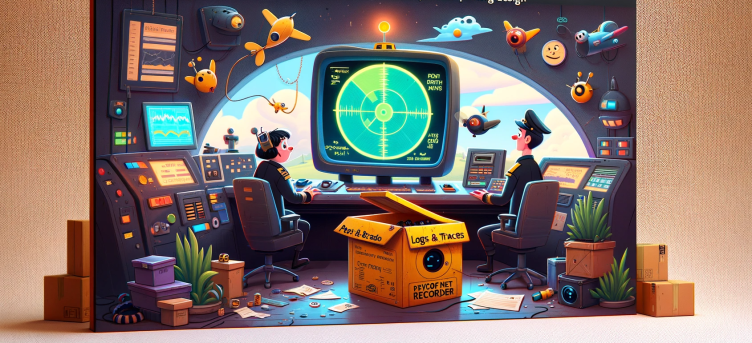
In a digital world, data powers everything from business decisions to customer experiences. But as data grows and becomes more complex, keeping track of its quality and reliability can be tough.
That’s where data observability steps in. It’s like a "health check" for your data, helping you catch issues early, ensure everything’s accurate, and keep trust in your data across all your systems.
What Is Data Observability?
Data observability is all about monitoring the health of your data throughout its lifecycle. It’s not just about collecting data; it’s about understanding how data flows through your systems, evolves over time, and impacts your business.
Unlike basic monitoring, which looks at system performance, data observability focuses on the quality, consistency, and behavior of your data.
When done right, it gives you a clear view of your data’s journey, helping you catch potential issues before they escalate and affect your decisions and outcomes.
Why Data Observability Is Essential for Your Business
Better Data Quality
Let’s face it: bad data leads to bad decisions. Data observability helps you ensure your data stays clean, accurate, and reliable, from start to finish. Catching issues early helps you avoid the headaches that come with working with incomplete or inaccurate data.
Catch Issues Early
Data systems can be complicated, and problems can be tough to spot—especially when they’re hidden deep in the data pipeline. Data observability lets you monitor things live, so when something goes off-track, you can step in quickly and prevent bigger issues down the line.
Build Trust in Your Data
In a world where data drives almost every decision, trust is everything. With data observability, you get a clear picture of how your data is handled—from where it comes from to how it’s transformed. This transparency not only boosts confidence in your data but also helps your team work together more smoothly.
Boost Team Collaboration
When everyone—whether they’re data engineers or analysts—has access to the same data health metrics, you create a shared understanding of how data behaves across systems. This makes it much easier to collaborate and resolve issues faster.
Prepare for Growth
As your company grows, so will your data needs. Data observability ensures that your data flow stays efficient and optimized, no matter how much more data you’re dealing with. It helps you stay ahead of the curve, so your systems keep performing well as you scale.
Key Components of Data Observability
To get the most out of data observability, you’ll need to focus on a few key elements:
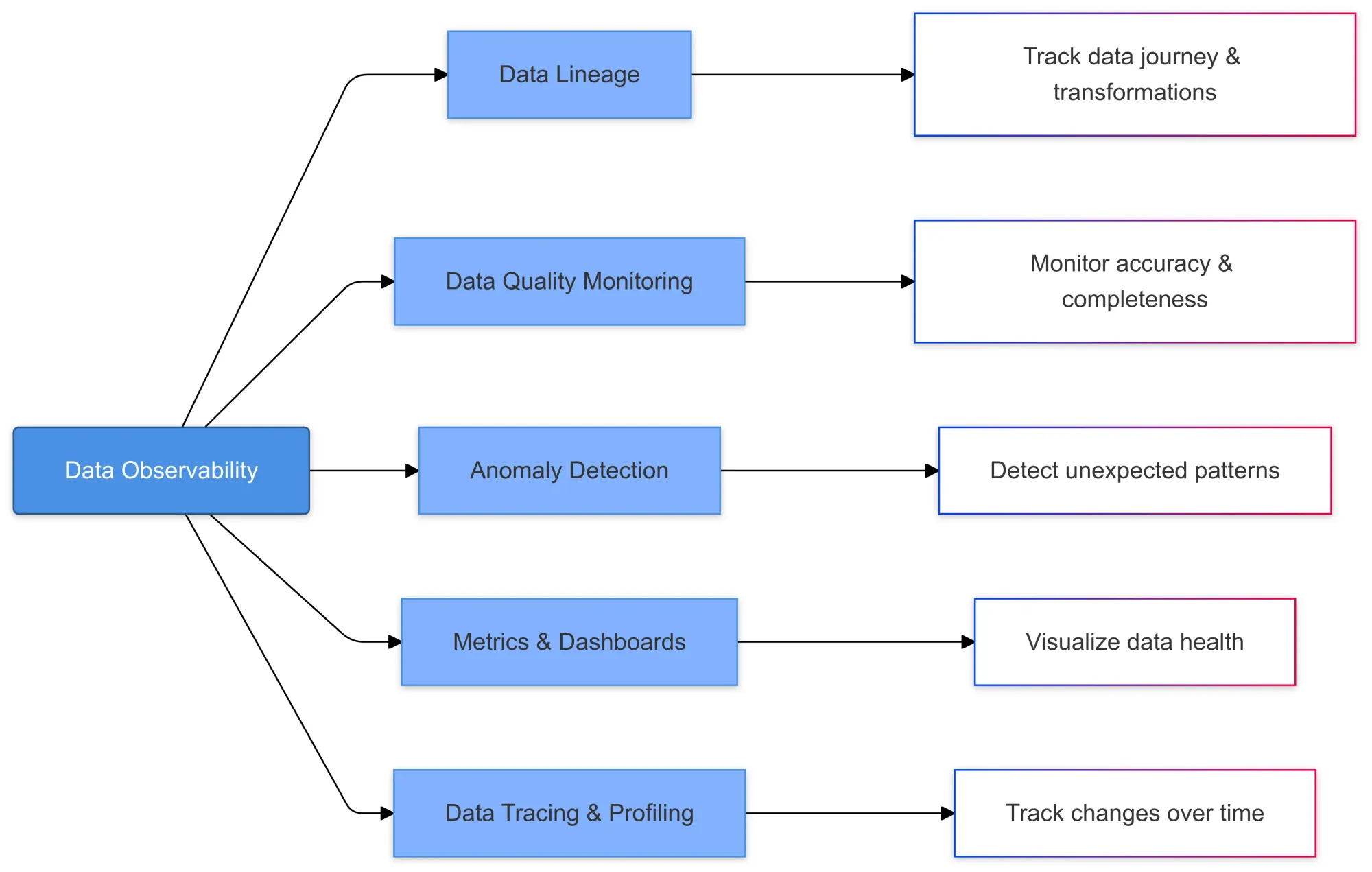
1. Data Lineage
Data lineage is all about tracking your data’s journey from start to finish. Knowing where your data comes from and how it’s transformed as it moves through systems makes it easier to troubleshoot issues and understand the impact of changes.
2. Data Quality Monitoring
Keeping track of data quality across the board is a must. Data observability helps you automate checks for accuracy, completeness, and timeliness. This way, you catch issues early and ensure you’re working with the best data possible.
3. Anomaly Detection
Data doesn’t always behave the way you expect it to. Whether it’s caused by changes in source systems or new user behaviors, anomalies can pop up at any time. Data observability tools use smart analytics to spot these odd patterns before they lead to bigger issues, helping you stay on top of things.
4. Metrics and Dashboards
Dashboards are where you get the full picture. These centralized views of your data health metrics let you see instantaneously how your data is performing. Whether it’s tracking freshness, volume, or consistency, dashboards keep you in the loop.
5. Data Tracing and Profiling
Profiling and tracing tools allow you to track how your data changes over time. This helps ensure consistency and spot any issues with data duplication or corruption early in the process.
How to Get Started with Data Observability
If you’re thinking about implementing data observability, here are a few tips to make sure you’re on the right track:
1. Have a Clear Plan
Before jumping in, take a step back and define your goals. Are you trying to improve data quality? Detect issues earlier? Build trust in your data? Knowing what you want to achieve will help you choose the right tools and approach.
2. Pick the Right Tools
There are a lot of observability tools out there, but not all of them will fit your needs. When choosing, look for platforms that integrate easily with your current systems and offer the key features you need, like anomaly detection, data lineage, and real-time monitoring.
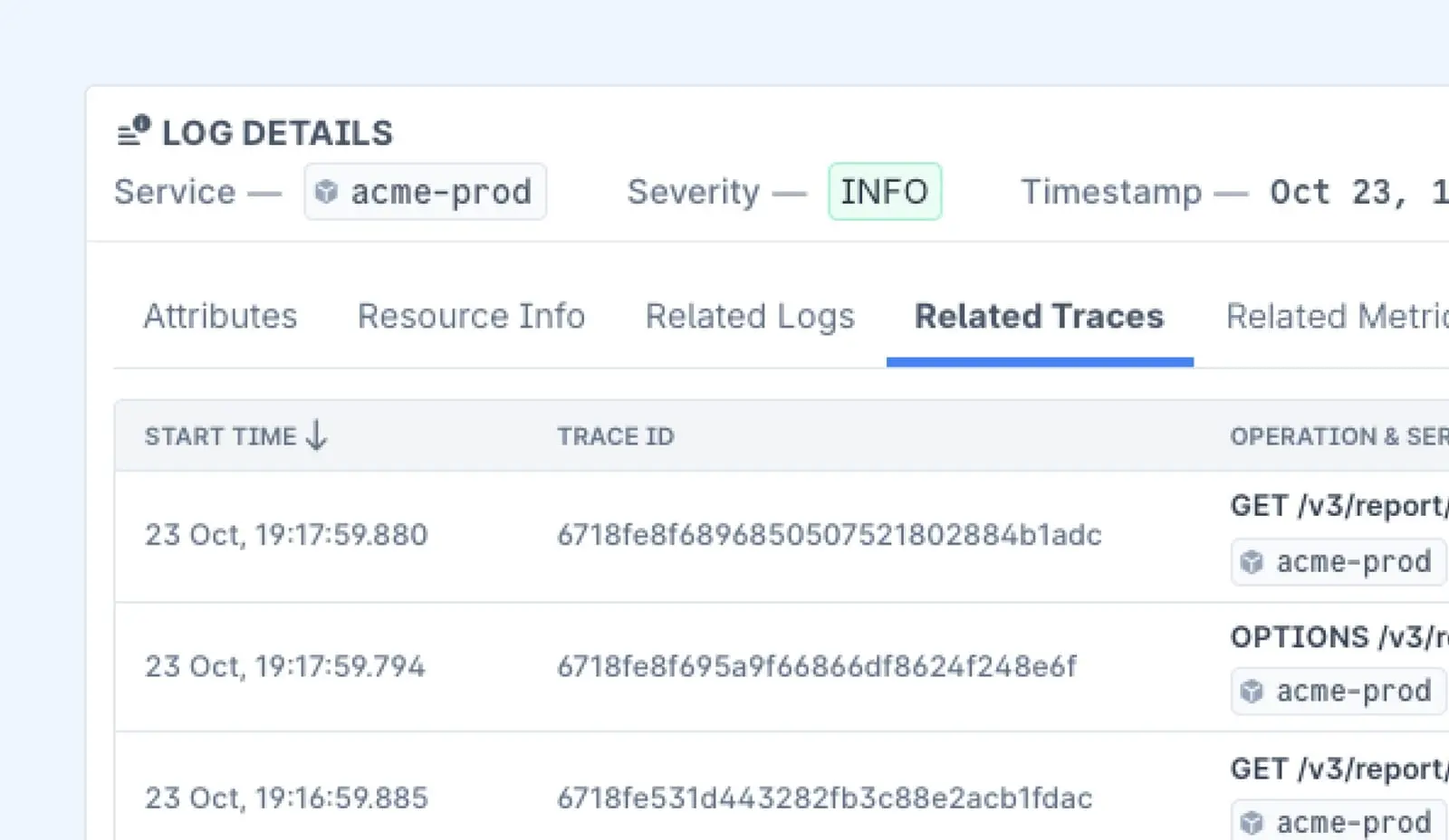
3. Automate What You Can
Data observability can get overwhelming if you’re manually checking everything. Automation is key. Set up automated alerts for data quality issues, performance problems, and anomalies to keep things running smoothly without constant oversight.
4. Create a Data-Centric Culture
Data observability isn’t just about the tools—it’s about fostering a culture that values data quality and transparency. Encourage cross-functional teams to collaborate and ensure everyone has the right visibility into your data’s health.
5. Keep Improving
Data observability is an ongoing effort. As your data environment grows and evolves, so should your observability practices. Regularly review your tools, processes, and metrics to keep up with changes and ensure your systems stay healthy.
The Key Benefits of Data Observability
As businesses continue to rely on data for decision-making, ensuring its accuracy, consistency, and reliability becomes increasingly crucial.
Data observability offers several advantages, not just in terms of detecting issues but also in creating a culture of proactive data management.
Below, we’ll explore the major benefits of embracing data observability and why it's worth the investment.
1. Improved Data Quality
Data quality is the foundation of good decision-making. Inaccurate, incomplete, or outdated data can lead to poor business outcomes and a loss of trust from stakeholders. Data observability helps you monitor your data across its entire lifecycle, enabling you to spot issues early.
Whether it's missing values, incorrect formats, or unexpected anomalies, you can catch these problems before they escalate.
With continuous monitoring in place, you can set up automated quality checks to validate that your data is correct, consistent, and up-to-date, ensuring that the information driving business decisions is always reliable.
2. Early Detection of Issues
The sooner you identify data problems, the less impact they’ll have on your operations.
In a traditional data pipeline, problems may not be evident until they’ve already caused significant issues, like incorrect reporting, misleading analytics, or even service disruptions. With data observability, you can set up alerts to detect issues instantaneously.
Identifying problems as they happen—whether it's an unexpected drop in data volume, slow data processing times, or changes in data patterns—lets you quickly address the root cause, minimizing downtime or disruptions to your workflow.
3. Increased Operational Efficiency
Operational efficiency is a critical factor in data-driven businesses. Data observability simplifies the process of managing data pipelines by automating many of the monitoring and quality checks that would otherwise require manual intervention.
With real-time dashboards, alerts, and reports, data teams can quickly prioritize and resolve issues that impact the health of the data systems. This increased visibility allows teams to focus on more strategic initiatives rather than spending time troubleshooting or manually inspecting data.
4. Improved Collaboration Across Teams
In many organizations, data is handled by multiple teams across different departments. Data engineers, data scientists, analysts, and business users often interact with the same datasets but have different goals, needs, and expectations.
Data observability breaks down silos by providing a single, unified view of the data across the organization. With a shared understanding of how data is performing, teams can collaborate more effectively.
For example, if an anomaly is detected, engineers can quickly address it, while analysts can explore the impact of the anomaly on reporting. This approach ensures that everyone is on the same page and working towards maintaining data integrity.
5. Better Decision-Making
Data observability empowers organizations to make informed, confident decisions based on high-quality, reliable data. With real-time visibility into data pipelines, teams can understand exactly how data is flowing, where it might be getting stuck, and whether the outputs are reliable.
When business leaders trust that the data they’re using to make decisions is accurate and complete, they can make faster, more informed choices.
For instance, marketing teams can confidently rely on customer data to adjust campaigns, while sales teams can trust the metrics they’re seeing when adjusting their sales strategies.
6. Faster Time-to-Market for New Initiatives
For businesses looking to innovate, time-to-market is often critical. Data observability speeds up the process of building and deploying new data products, services, and features by ensuring that data flows efficiently and accurately.
When you have a real-time view of your data’s health, you can identify and address any issues that may delay the launch of new initiatives. Whether it’s a new product feature, a reporting dashboard, or an AI model, data observability allows you to move faster and with greater confidence.
7. Increased Trust in Data
Trust is a crucial element of any data-driven organization. When data is unreliable or inconsistent, teams lose faith in it, which leads to confusion, delays, and a reluctance to use it for decision-making.
With robust data observability in place, you create an environment of transparency, where data quality is continuously monitored, and issues are proactively addressed.
Being transparent about the health of your data and showing a clear trail of how it’s tracked, cleaned, and validated increases trust within the organization and with external stakeholders.
8. Greater Scalability and Adaptability
As businesses grow, their data needs evolve. Data observability helps ensure that your data infrastructure is capable of handling increased data volumes, new data sources, and new types of analytics.
Whether you're scaling up your data pipelines or integrating new systems, observability tools can help you identify bottlenecks and optimize performance.
Data observability also makes it easier to adapt to changes, such as the introduction of new data sources or shifts in business priorities.
Keeping an eye on your data live allows you to more effectively adjust your processes and systems to accommodate growth.
9. Improved Compliance and Risk Management
Data privacy and regulatory compliance are top concerns for many organizations, especially as they collect and process more data.
With data observability, you can track data lineage, ensuring that you know where your data comes from, how it’s transformed, and where it goes. This level of visibility is key for meeting compliance standards and reducing risk.
Whether it’s adhering to GDPR, HIPAA, or other data protection regulations, data observability tools help ensure that data is handled correctly and that you’re able to quickly respond to any compliance-related issues.
10. Cost Savings in the Long Run
While implementing data observability tools and practices may require an upfront investment, the long-term cost savings are significant.
Identifying issues early and improving data quality helps you avoid the costly consequences of bad data, like wasted resources, inaccurate business strategies, or system downtime.
Additionally, the operational efficiencies gained through automated monitoring and real-time alerting reduce the burden on your team, lowering costs associated with troubleshooting and data remediation.
Ultimately, data observability allows you to spend less time fixing issues and more time driving value from your data.
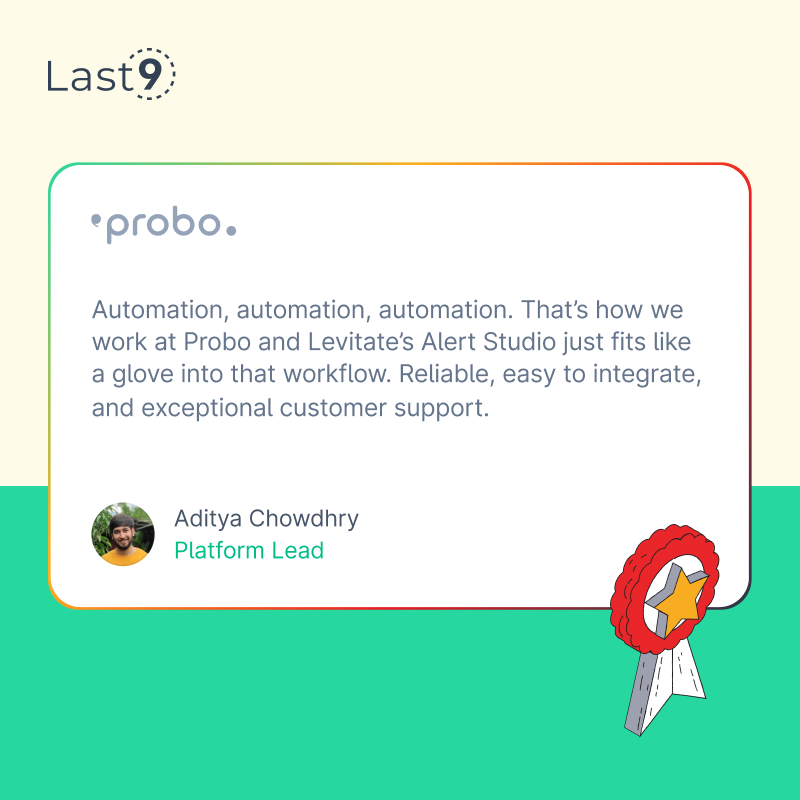
Data Observability vs. Other Concepts: Understanding the Differences
As the world of data continues to grow, it's easy to get lost in the different terms and tools used to ensure that your data is functioning well.
In this section, we’ll break down the key differences between data observability and related concepts like data quality, data governance, data monitoring, and data testing.
1. Data Observability vs. Data Quality
Data quality is all about ensuring that the data you collect is accurate, consistent, reliable, and timely. In simpler terms, data quality focuses on the “health” of your data at any given moment. It’s about making sure your data meets certain standards and is fit for use in decision-making.
On the other hand, data observability takes a more proactive approach. While data quality deals with ensuring that data is correct, data observability monitors the entire data pipeline to track the health of the data as it moves, changes, and evolves.
It's like a watchful guardian, keeping an eye on your data’s journey across various systems, ensuring you can detect any disruptions or quality issues before they become significant problems.
In short: Data quality ensures your data is good, while data observability helps you track and maintain that quality across the data lifecycle.
2. Data Observability vs. Data Governance
Data governance refers to the policies, procedures, and practices put in place to manage data assets effectively and securely. This includes setting up rules for who can access the data, how it should be stored, and how data privacy and compliance should be handled.
The goal of data governance is to ensure that data is used responsibly and legally within an organization.
While data observability shares some overlap with data governance (since it helps ensure data is monitored and handled correctly), its focus is more on tracking the behavior and health of the data.
Data governance lays the foundation for how data should be managed, while data observability provides the tools to ensure data is being tracked, monitored, and remains reliable as it flows through different systems.
In short: Data governance sets the rules for managing data; data observability helps you monitor and ensure those rules are followed and the data behaves as expected.
3. Data Observability vs. Data Monitoring
Data monitoring involves continuously checking data pipelines for performance, identifying failures, and ensuring data systems are functioning as expected.
Monitoring tools track metrics like uptime, processing speed, and system performance, offering alerts when issues arise.
However, data observability goes beyond monitoring by not just tracking performance metrics but also diving into the underlying behavior of your data.
It enables you to detect not just when something is broken but also why it’s happening—whether that’s an issue with data integrity, pipeline disruptions, or unforeseen anomalies.
In short: Data monitoring tracks performance and alerts you when things go wrong, while data observability provides deep insights into why things go wrong and helps you understand your data’s behavior across the entire system.
4. Data Observability vs. Data Testing
Data testing focuses on verifying the correctness of data, especially when it's being processed or transformed. It involves validating that the data is accurate and conforms to predefined rules or expectations.
Testing ensures that your data pipeline is working as expected, which is crucial for preventing errors from reaching production. While data testing is a key aspect of data observability, data observability encompasses much more.
Observability tracks your data’s health continuously, offering insights into potential issues, patterns, and anomalies as they arise. It goes beyond testing and provides visibility into the entire data lifecycle, even when you aren't actively testing data.
In short: Data testing ensures specific pieces of data are correct at a point in time, while data observability provides ongoing insights into data health and system behavior.
Key Differences at a Glance
Concept | Focus | Scope |
---|---|---|
Data Observability | Tracking data health throughout the entire lifecycle. | Provides visibility into data behavior, quality, and system status in real-time. |
Data Quality | Ensuring data accuracy, consistency, and timeliness. | Focuses on the correctness and reliability of data. |
Data Governance | Managing data assets and ensuring compliance and security. | Focuses on policies and practices around data access and security. |
Data Monitoring | Tracking performance metrics and system uptime. | Provides alerts for performance issues, without diving into data specifics. |
Data Testing | Verifying data correctness at a specific point in time. | Ensures data transformation and validation are correct. |
Data Observability Challenges to Consider
While data observability can bring huge benefits, there are a few challenges you might face along the way:
- Complex Data Environments: Managing observability across a mix of on-prem and cloud-based systems can be tricky. Make sure the tools you choose can handle this complexity.
- Data Silos: Data often gets locked away in different departments or systems, making it hard to get a full view of data health. Breaking down these silos is essential for effective observability.
- Skills Shortage: Data observability requires specialized knowledge, and not all organizations have the right resources on hand. Training your team or hiring new talent might be necessary to build a strong observability framework.
Wrapping Up
With the right tools, strategy, and a focus on continuous improvement, data observability can help you stay ahead of issues, scale your data infrastructure, and build a more data-driven organization.
FAQs
What is data observability?
Data observability is the practice of monitoring and understanding data health throughout its lifecycle, ensuring quality, consistency, and business impact.
How does data observability differ from data quality?
Data observability tracks the overall health and flow of data, while data quality focuses on accuracy, consistency, and reliability at a given moment.
Why is data observability important for businesses?
It helps identify data issues before they affect decisions, ensuring data is accurate and aligned with business goals to prevent costly errors.
How can data observability improve decision-making?
It provides real-time insights, ensuring decision-makers have access to clean, accurate, and up-to-date information.
What tools are used for data observability?
Tools like Monte Carlo, Bigeye, and Databand track data health, offering features like real-time monitoring, data lineage, and anomaly detection.
How does data observability help with data reliability?
It helps identify and address issues early, leading to higher reliability and fewer disruptions in data systems.
Can data observability help with scaling?
Yes, it provides insights into data flow, helping systems scale smoothly and preventing bottlenecks as data volume increases.
How is data observability different from data monitoring?
Data observability offers deep insights into data quality and flow, while monitoring focuses on system performance and issue alerts.
What are the benefits of implementing data observability?
Improved data quality, earlier issue detection, better collaboration, and increased trust in data lead to better decision-making and outcomes.
Can data observability help prevent data downtime?
Yes, it helps catch issues early, providing visibility that prevents downtime or disruptions caused by data quality or system problems.
Contents
Newsletter
Stay updated on the latest from Last9.
Helping to make the tech a little less intimidating. I love breaking down complex concepts into easy-to-understand terms.
Handcrafted Related Posts
The Practical Guide to Alert Sanity: From Chaos to Calm
Your pager just went off. Is it the CPU again? Memory? Disk space? Wrong question. Ask: Can users do their thing? That's your real alert.
Aditya Godbole
Doing SRE the Right Way!
A well-thought-out approach to SRE, which will help site reliability engineers and software engineers develop and maintain a useful, consistent, and effective SRE strategy for their products!
Piyush Verma
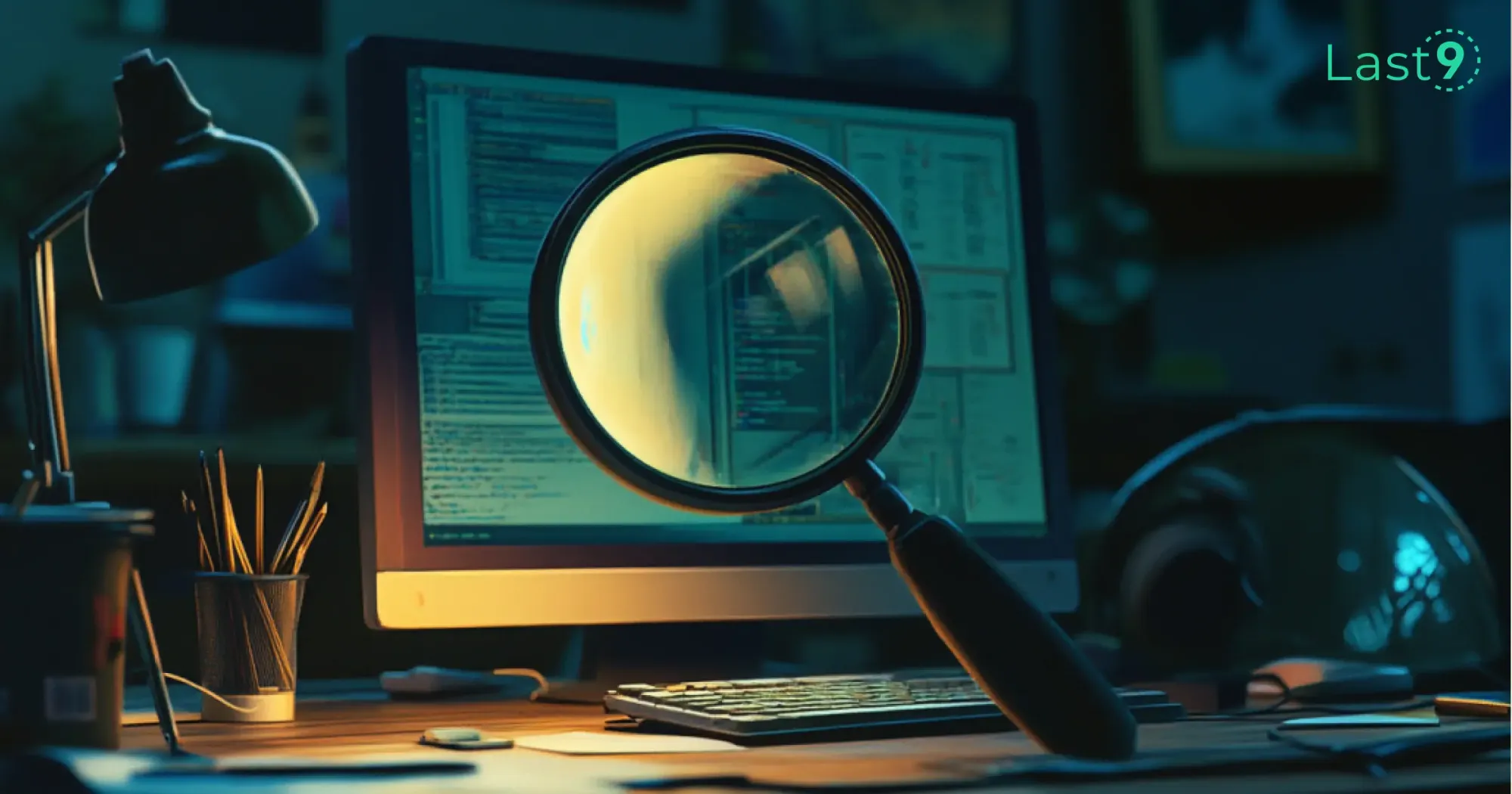
The Ultimate Guide to Application Performance Monitoring (APM)
Learn everything about Application Performance Monitoring (APM), from its definition to its crucial role in optimizing application performance.
Anjali Udasi