When React applications grow in complexity, understanding performance bottlenecks and tracking down issues becomes increasingly difficult. Developers often face challenges identifying slow API calls, component rendering delays, or understanding how user interactions flow through the application stack.
OpenTelemetry offers a standardized approach to gaining visibility into your React applications. This guide walks through the implementation process with practical examples and solutions to common challenges that developers face when adding observability to their frontend applications.
What is OpenTelemetry?
OpenTelemetry (often abbreviated as OTel) is an open-source observability framework that helps you collect telemetry data—metrics, logs, and traces—from your applications. Think of it as the standardized plumbing that connects your app to whatever monitoring system you prefer.
The beauty of OpenTelemetry? Write the instrumentation once, then send that data to any compatible backend. No vendor lock-in.
Why Should React Developers Care About OpenTelemetry?
React makes building UIs easier, but when things go wrong, figuring out what happened can be challenging. With proper OpenTelemetry instrumentation, developers can:
- Track user interactions and how they cascade through the application
- Identify slow components and excessive re-renders
- Measure API call performance from the frontend perspective
- Correlate backend issues with frontend experiences
This creates a trail of breadcrumbs throughout the entire application stack.
How Do You Set Up OpenTelemetry in Your React App?
This section walks through the essential steps to add OpenTelemetry to a React application.
Step 1: Install the Required Packages
First, install the necessary packages:
npm install @opentelemetry/api @opentelemetry/sdk-trace-web @opentelemetry/context-zone @opentelemetry/instrumentation @opentelemetry/instrumentation-document-load @opentelemetry/instrumentation-fetch @opentelemetry/instrumentation-xml-http-request @opentelemetry/exporter-trace-otlp-http
Step 2: Create a Telemetry Configuration
Create a new file called telemetry.js
in your project:
import { WebTracerProvider } from '@opentelemetry/sdk-trace-web';
import { registerInstrumentations } from '@opentelemetry/instrumentation';
import { ZoneContextManager } from '@opentelemetry/context-zone';
import { FetchInstrumentation } from '@opentelemetry/instrumentation-fetch';
import { XMLHttpRequestInstrumentation } from '@opentelemetry/instrumentation-xml-http-request';
import { DocumentLoadInstrumentation } from '@opentelemetry/instrumentation-document-load';
import { BatchSpanProcessor } from '@opentelemetry/sdk-trace-base';
import { OTLPTraceExporter } from '@opentelemetry/exporter-trace-otlp-http';
import { Resource } from '@opentelemetry/resources';
import { SemanticResourceAttributes } from '@opentelemetry/semantic-conventions';
export const setupTelemetry = () => {
const resource = new Resource({
[SemanticResourceAttributes.SERVICE_NAME]: 'react-app',
[SemanticResourceAttributes.SERVICE_VERSION]: '1.0.0',
});
const provider = new WebTracerProvider({ resource });
// Create and configure OTLP exporter
const otlpExporter = new OTLPTraceExporter({
url: 'http://localhost:4318/v1/traces', // Update with your collector endpoint
});
// Use BatchSpanProcessor for better performance
const spanProcessor = new BatchSpanProcessor(otlpExporter);
provider.addSpanProcessor(spanProcessor);
// Register the provider
provider.register({
contextManager: new ZoneContextManager(),
});
// Register instrumentations
registerInstrumentations({
instrumentations: [
new DocumentLoadInstrumentation(),
new FetchInstrumentation({
// Ignore certain URLs from being instrumented
ignoreUrls: [/localhost:8090\/sockjs-node/],
// Add custom headers to your outgoing requests
propagateTraceHeaderCorsUrls: [
/.+/g, // Propagate to all URLs, for demo purposes
],
}),
new XMLHttpRequestInstrumentation({
propagateTraceHeaderCorsUrls: [
/.+/g, // Propagate to all URLs, for demo purposes
],
}),
],
});
};
Step 3: Initialize OpenTelemetry in Your App
Update your index.js
or main.jsx
file to initialize OpenTelemetry before your app renders:
import React from 'react';
import ReactDOM from 'react-dom';
import App from './App';
import { setupTelemetry } from './telemetry';
// Initialize OpenTelemetry
setupTelemetry();
ReactDOM.render(
<React.StrictMode>
<App />
</React.StrictMode>,
document.getElementById('root')
);
Step 4: Create Custom Spans for React Components
Let's add some custom instrumentation to track component performance:
import { trace } from '@opentelemetry/api';
import React, { useEffect } from 'react';
const tracer = trace.getTracer('react-components');
function ProductList({ products }) {
useEffect(() => {
const span = tracer.startSpan('ProductList.render');
// End the span when the component unmounts
return () => {
span.end();
};
}, []);
return (
<div>
<h2>Products</h2>
<ul>
{products.map(product => (
<li key={product.id}>{product.name} - ${product.price}</li>
))}
</ul>
</div>
);
}
export default ProductList;
How Can You Gather Meaningful Metrics from React Apps?
With a basic tracing setup, the focus shifts to metrics that matter for React applications.
React Render Performance
Here's how to track component render times:
import { trace } from '@opentelemetry/api';
import React, { useEffect, useState } from 'react';
const tracer = trace.getTracer('react-components');
function useComponentTracer(componentName) {
useEffect(() => {
const span = tracer.startSpan(`${componentName}.lifecycle`);
// Record the mount event
span.addEvent('component.mounted');
return () => {
// Record the unmount event
span.addEvent('component.unmounted');
span.end();
};
}, [componentName]);
}
function ExpensiveComponent({ data }) {
useComponentTracer('ExpensiveComponent');
const [processed, setProcessed] = useState(null);
useEffect(() => {
const span = tracer.startSpan('ExpensiveComponent.dataProcessing');
// Simulate expensive data processing
const processData = () => {
const start = performance.now();
// Actual processing logic would go here
const result = data.map(item => ({ ...item, processed: true }));
const end = performance.now();
span.setAttribute('processing.time_ms', end - start);
span.end();
setProcessed(result);
};
processData();
}, [data]);
return processed ? (
<div>
<h3>Processed {processed.length} items</h3>
{/* Render your processed data */}
</div>
) : (
<div>Processing data...</div>
);
}
User Interactions
Track how users interact with your application:
import { trace, context } from '@opentelemetry/api';
import React from 'react';
const tracer = trace.getTracer('user-interactions');
function SearchBar() {
const handleSearch = (event) => {
const searchTerm = event.target.value;
// Create a span for the search operation
const span = tracer.startSpan('user.search');
span.setAttribute('search.term', searchTerm);
// Use the context API to bind the current context
const ctx = trace.setSpan(context.active(), span);
context.with(ctx, () => {
// Any async operations started here will be properly
// associated with the parent span
fetchSearchResults(searchTerm).finally(() => {
span.end();
});
});
};
return (
<input
type="text"
placeholder="Search..."
onChange={handleSearch}
/>
);
}
async function fetchSearchResults(term) {
// This function will automatically create a child span
// due to the fetch instrumentation we set up earlier
const response = await fetch(`/api/search?q=${term}`);
return response.json();
}
How Do You Connect Frontend to Backend Traces?
One of the most powerful features of OpenTelemetry is the ability to connect frontend and backend traces. This provides a complete picture of the user experience.
Propagating Context in API Calls
import { trace, context, propagation } from '@opentelemetry/api';
import React, { useState, useEffect } from 'react';
const tracer = trace.getTracer('api-client');
async function fetchWithTracing(url, options = {}) {
const span = tracer.startSpan('api.request');
span.setAttribute('http.url', url);
try {
// Add the current trace context to the headers
const headers = options.headers || {};
const newHeaders = { ...headers };
propagation.inject(context.active(), newHeaders);
const response = await fetch(url, {
...options,
headers: newHeaders,
});
span.setAttribute('http.status_code', response.status);
return response;
} catch (error) {
span.setAttribute('error', true);
span.setAttribute('error.message', error.message);
throw error;
} finally {
span.end();
}
}
function UserProfile({ userId }) {
const [user, setUser] = useState(null);
const [loading, setLoading] = useState(true);
const [error, setError] = useState(null);
useEffect(() => {
const loadUser = async () => {
try {
const response = await fetchWithTracing(`/api/users/${userId}`);
const data = await response.json();
setUser(data);
} catch (err) {
setError(err.message);
} finally {
setLoading(false);
}
};
loadUser();
}, [userId]);
if (loading) return <div>Loading...</div>;
if (error) return <div>Error: {error}</div>;
return (
<div>
<h2>{user.name}</h2>
<p>Email: {user.email}</p>
{/* Other user details */}
</div>
);
}
What Are Common React OpenTelemetry Issues and How Do You Fix Them?
Even with careful setup, issues can arise. Here's how to fix the most common problems:
No Spans Appearing in the Backend
The Problem: OpenTelemetry is set up, but no data appears in the observability tool.
Solutions:
- Check CORS Configuration: Many backends reject cross-origin requests. Make sure your collector or backend allows requests from your React app's domain.
// In your telemetry.js file
const otlpExporter = new OTLPTraceExporter({
url: 'http://localhost:4318/v1/traces',
headers: {}, // Add any required auth headers
});
- Verify Your Endpoint: Double-check that the endpoint URL is correct and accessible from your browser.
- Look for Console Errors: Check your browser's console for any error messages related to the OpenTelemetry exports.
Memory Leaks from Unclosed Spans
The Problem: Your app's memory usage grows over time because spans aren't being closed properly.
Solution: Always close spans, especially in components that might unmount:
function ComponentWithCleanup() {
useEffect(() => {
const span = tracer.startSpan('component.lifecycle');
// Return cleanup function to end span on unmount
return () => {
span.end();
};
}, []);
// Component content
}
Too Many Spans Being Generated
The Problem: Your app is generating too many spans, causing performance issues or exceeding backend limits.
Solution: Be selective about what you instrument:
// Only create spans for specific components or actions
function ShouldCreateSpan(componentName) {
// List of components we want to trace
const tracedComponents = ['ExpensiveComponent', 'SearchResults', 'UserProfile'];
return tracedComponents.includes(componentName);
}
function Component({ name }) {
useEffect(() => {
// Only create spans for important components
if (ShouldCreateSpan(name)) {
const span = tracer.startSpan(`${name}.lifecycle`);
return () => span.end();
}
}, [name]);
// Component content
}
How Do You Choose the Right Observability Backend?
With OpenTelemetry, data can be sent to various backends. Here's a comparison to help with the selection process:
Last9
Best for: High-cardinality observability at scale and full-stack observability
Setup Complexity: Low
Cost Model: Based on the number of events ingested
For those looking for a budget-friendly, managed observability solution without compromising performance, Last9 is an ideal choice. As a telemetry data platform, we’ve successfully monitored 11 of the 20 largest live-streaming events in history, showcasing our ability to handle massive scale.
Last9 integrates seamlessly with OpenTelemetry and Prometheus, centralizing metrics, logs, and traces into one unified platform. This comprehensive view optimizes performance monitoring, cost management, and real-time insights with correlated monitoring and alerting. Plus, with Last9 MCP, you can bring real-time production context — logs, metrics, and traces — into your local environment, helping you auto-fix code faster.
Trusted by companies like Probo, CleverTap, and Replit for managing high-cardinality data, Last9 ensures you can stay on top of your observability needs.
Jaeger
Best for: Local development and testing
Setup Complexity: Medium
Cost Model: Free (self-hosted)
Jaeger is a powerful, open-source distributed tracing system designed for developers working in local or test environments.
While it’s well-suited for tracing complex microservices and observing latency, Jaeger requires a self-hosted setup, which can involve moderate configuration. Its lightweight nature makes it ideal for debugging during development, but it may require additional infrastructure for large-scale use.
Zipkin
Best for: Simple distributed tracing
Setup Complexity: Low
Cost Model: Free (self-hosted)
Zipkin is another open-source solution focused on distributed tracing, offering a simple and effective way to track requests across services. It's known for its easy setup and low overhead, making it a go-to for teams seeking quick, straightforward trace analysis without the need for complex configuration. However, like Jaeger, it's self-hosted, which can limit its scalability in larger environments.
Grafana Tempo
Best for: Integration with the Grafana ecosystem
Setup Complexity: Medium
Cost Model: Free + paid options
Grafana Tempo is a robust tracing backend that integrates seamlessly with the Grafana ecosystem, providing an excellent choice for teams already using Grafana for monitoring and dashboards.
With both free and paid tiers, it’s a flexible solution that can scale alongside your needs, whether you're monitoring a small app or managing a large-scale system. Its ease of use and native Grafana integration make it ideal for teams familiar with the Grafana suite.
Datadog
Best for: Full-stack observability and unified monitoring
Setup Complexity: Medium
Cost Model: Pay per host/metric
Datadog is a popular choice for teams that need a comprehensive observability solution that integrates metrics, logs, traces, and more in one platform. It’s widely used for full-stack monitoring, providing deep insights into system performance and user behavior.
While it requires a bit more configuration compared to simpler tools, Datadog’s extensive features and integrations make it well-suited for large, complex environments. Its pricing is based on the number of hosts or metrics, so it scales with your infrastructure.
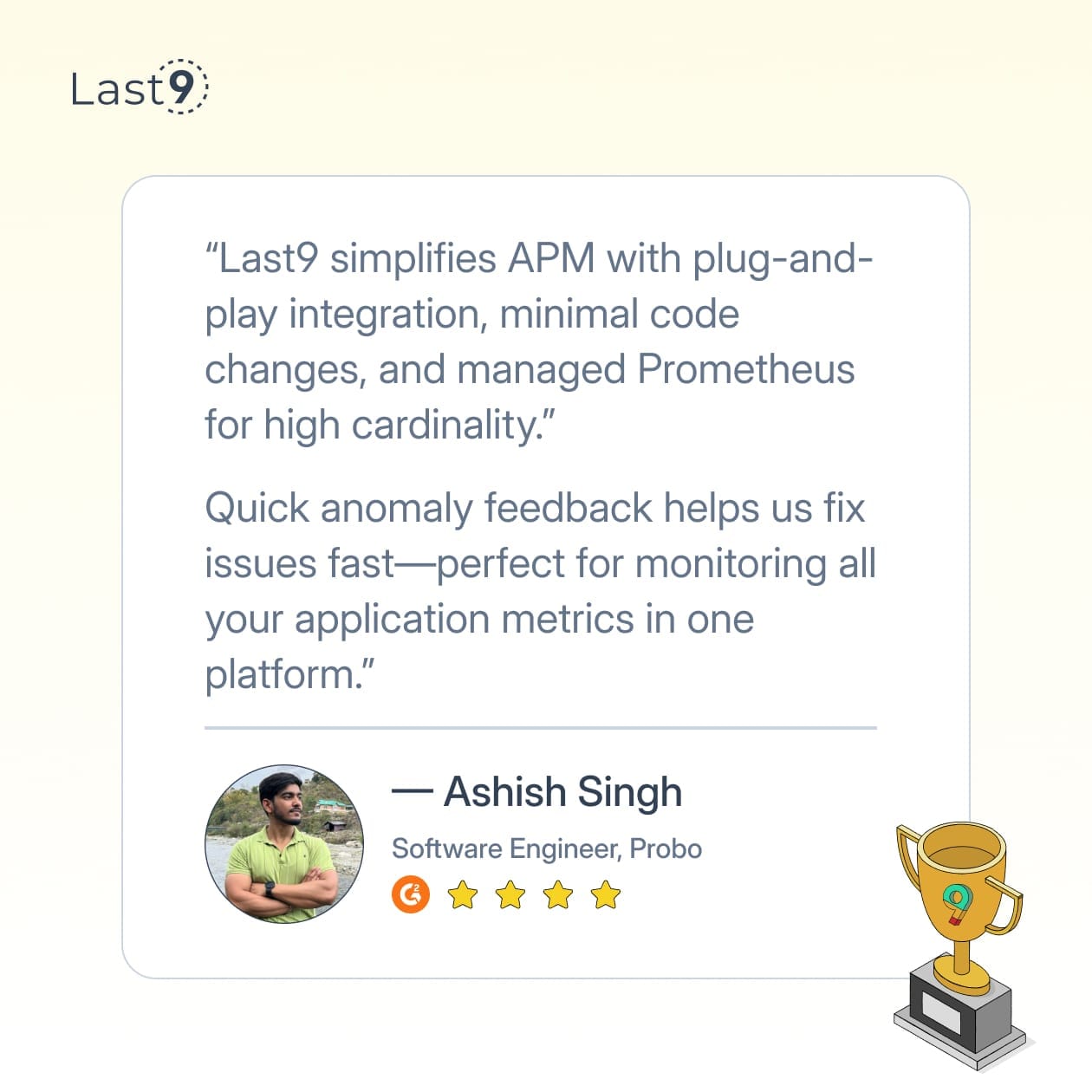
Advanced Techniques You Can Use Beyond Basic Tracing
Once the basics are established, consider these more advanced techniques:
Custom Error Boundaries with Telemetry
import React, { Component } from 'react';
import { trace } from '@opentelemetry/api';
const tracer = trace.getTracer('error-handling');
class TelemetryErrorBoundary extends Component {
constructor(props) {
super(props);
this.state = { hasError: false, error: null };
}
static getDerivedStateFromError(error) {
return { hasError: true, error };
}
componentDidCatch(error, errorInfo) {
// Record the error in telemetry
const span = tracer.startSpan('React.ErrorBoundary');
span.setAttribute('error', true);
span.setAttribute('error.message', error.message);
span.setAttribute('error.stack', error.stack || '');
span.setAttribute('error.componentStack', errorInfo.componentStack);
span.end();
// You could also send this to your error reporting service
}
render() {
if (this.state.hasError) {
return this.props.fallback || <h2>Something went wrong.</h2>;
}
return this.props.children;
}
}
// Usage
function App() {
return (
<TelemetryErrorBoundary fallback={<div>Oops! We're fixing this.</div>}>
<YourComponent />
</TelemetryErrorBoundary>
);
}
Performance Marks for Critical User Journeys
import { trace } from '@opentelemetry/api';
import React, { useState } from 'react';
const tracer = trace.getTracer('user-journeys');
function CheckoutFlow() {
const [step, setStep] = useState('cart');
const [journeySpan, setJourneySpan] = useState(null);
// Start the journey when component mounts
React.useEffect(() => {
const span = tracer.startSpan('user.checkout.journey');
span.addEvent('journey.started', { step: 'cart' });
setJourneySpan(span);
// End the journey span when component unmounts
return () => {
if (step !== 'complete') {
span.addEvent('journey.abandoned', { last_step: step });
}
span.end();
};
}, []);
const moveToNextStep = (currentStep, nextStep) => {
if (journeySpan) {
journeySpan.addEvent('step.completed', { step: currentStep });
journeySpan.addEvent('step.started', { step: nextStep });
}
setStep(nextStep);
};
// Render different steps based on current state
switch (step) {
case 'cart':
return (
<div>
<h2>Your Cart</h2>
<button onClick={() => moveToNextStep('cart', 'shipping')}>
Proceed to Shipping
</button>
</div>
);
case 'shipping':
return (
<div>
<h2>Shipping Information</h2>
<button onClick={() => moveToNextStep('shipping', 'payment')}>
Proceed to Payment
</button>
</div>
);
case 'payment':
return (
<div>
<h2>Payment</h2>
<button onClick={() => {
moveToNextStep('payment', 'complete');
journeySpan?.addEvent('journey.completed');
}}>
Complete Order
</button>
</div>
);
case 'complete':
return <h2>Order Complete! Thank you.</h2>;
default:
return null;
}
}
Conclusion
Adding OpenTelemetry to a React application provides visibility into what's happening in production. From tracking down performance issues to understanding user journeys, proper instrumentation makes troubleshooting easier when things go wrong.
FAQs
How much overhead does OpenTelemetry add to a React app?
When properly configured, OpenTelemetry adds minimal overhead—typically less than 1% performance impact. The batch processor helps by sending data in groups rather than one by one. If performance issues are noticed, sampling traces instead of collecting everything can help.
Can OpenTelemetry work with React Native?
Yes, but with some caveats. React Native requires different instrumentation packages. @opentelemetry/sdk-trace-base
should be used instead of the web-specific packages, and the export needs to be handled differently since React Native doesn't run in a browser context.
How do developers track Redux actions with OpenTelemetry?
A Redux middleware can be created that generates spans for each action:
import { trace } from '@opentelemetry/api';
const tracer = trace.getTracer('redux');
const telemetryMiddleware = store => next => action => {
const span = tracer.startSpan(`redux.action.${action.type}`);
span.setAttribute('action.type', action.type);
span.setAttribute('action.payload', JSON.stringify(action.payload));
try {
const result = next(action);
span.end();
return result;
} catch (error) {
span.setAttribute('error', true);
span.setAttribute('error.message', error.message);
span.end();
throw error;
}
};
// Add this to your Redux store setup
const store = createStore(
rootReducer,
applyMiddleware(telemetryMiddleware, /* other middleware */)
);
Does OpenTelemetry work with Server-Side Rendering (SSR)?
Yes, but a slightly different setup is required. For Next.js or similar frameworks, OpenTelemetry should be initialized in both the client and server environments, with appropriate configuration for each.
How can frontend and backend traces be correlated?
The propagation API automatically adds trace context to instrumented HTTP requests. The backend should also use OpenTelemetry and be configured to extract this context from incoming requests. The W3C Trace Context specification defines how this context is passed between systems.