When you ask the Engineering team to define Reliability, instead of the Product or Customer-Success teams, you only get the inside view of the system. Modern systems don’t go down. They degrade; mainly a fraction of workflows, impacting a narrow set of users or use cases.
These alleys and bylanes cannot be defined by Engineering. It has to be the Product or Customer Success teams. They are the ones who put a cost on the users of these workflows. To Engineering teams, all workflows are alike.
For example, a product supports both the Write and Read paths. The system does 90% writes, and 10% reads.
Everything should work 100% of the time. But in a business at scale, there will always be something not right. You triage/decide to ignore or attend to it.
Let’s see it from each department’s hat. How would they go about triaging, and what parameters would they consider?
How would Engineering triage?
- Because the Writes are more often than Reads, if a read path is degrading, it’s Priority 2 (P2).
- Or, if a read fails, it’s rare and must be a Priority 1 (P1).
But, this lacks any context and is stateless. And the onus of triaging on Engineering also burns the candle at both ends. Either they triage + meet Sprint goals. Or they miss Sprints and planned features. 😵💫
Remember, interruptions and triaging cannot be estimated ahead of time in a card. So adding them as a planned activity in your sprint is unfeasible.
Effective triaging needs an additional triaging parameter, which is not cause-oriented but rather impact-oriented.
This is also why we add a hostname to our instrumentation; to know where the problem originates. Also, why fields like environment, cluster, etc, are essential.
But, the corresponding fields to measure Impact are absent. Fields like Feature, Account, Customer, etc., are equally important to define, “Where is the impact?” By knowing who is impacted, Customer Success and Product teams can help triage better.
How would Customer Success triage?
- Customer Acme’s Read flow is impacted, but they’re a pilot customer and have not signed a Service Level Agreement (SLA). They can absorb 1% degradation.
- Or; Customer FooStudios is impacted and pays 75% of our Americas revenue. Wake up, everyone!
How would Product triage?
- Customer FooStudios’s “order-Coffee” feature is impacted. But it’s still in closed alpha. They sure can absorb some number of degradations.
- Or; our GA feature is degraded across 5% of customers. Wake up!
Understanding Reliability
The definition of Reliability is not local to a single team. The same property should be dissected to know the impact.
- Engineering tries to know the cause.
- Product Management tries to know the impacted feature.
- Customer Success tries to know the impacted customer.
Instrumentation of Observability
The instrumentation must be solid — where is the information when needed the most? Sadly, or luckily, this data has to be instrumented. The possible axis, or dimensions, must be present in the instrumentation. And, that is an Engineering problem that needs a sprint or few.
Cardinality and Scale in Queryability
These dimensions are what we call Cardinality in Instrumentation. The underlying storage system must be capable of handling this diversity of information. The problem with most managed service offerings is, Observability is defined for a particular team. This automatically limits the answers you can extract.
So, what choice do you have?
Levitate, by Last9, was designed to solve the problems of Cardinality and Scale from the ground up. How does this work, you ask? This should give you some answers...
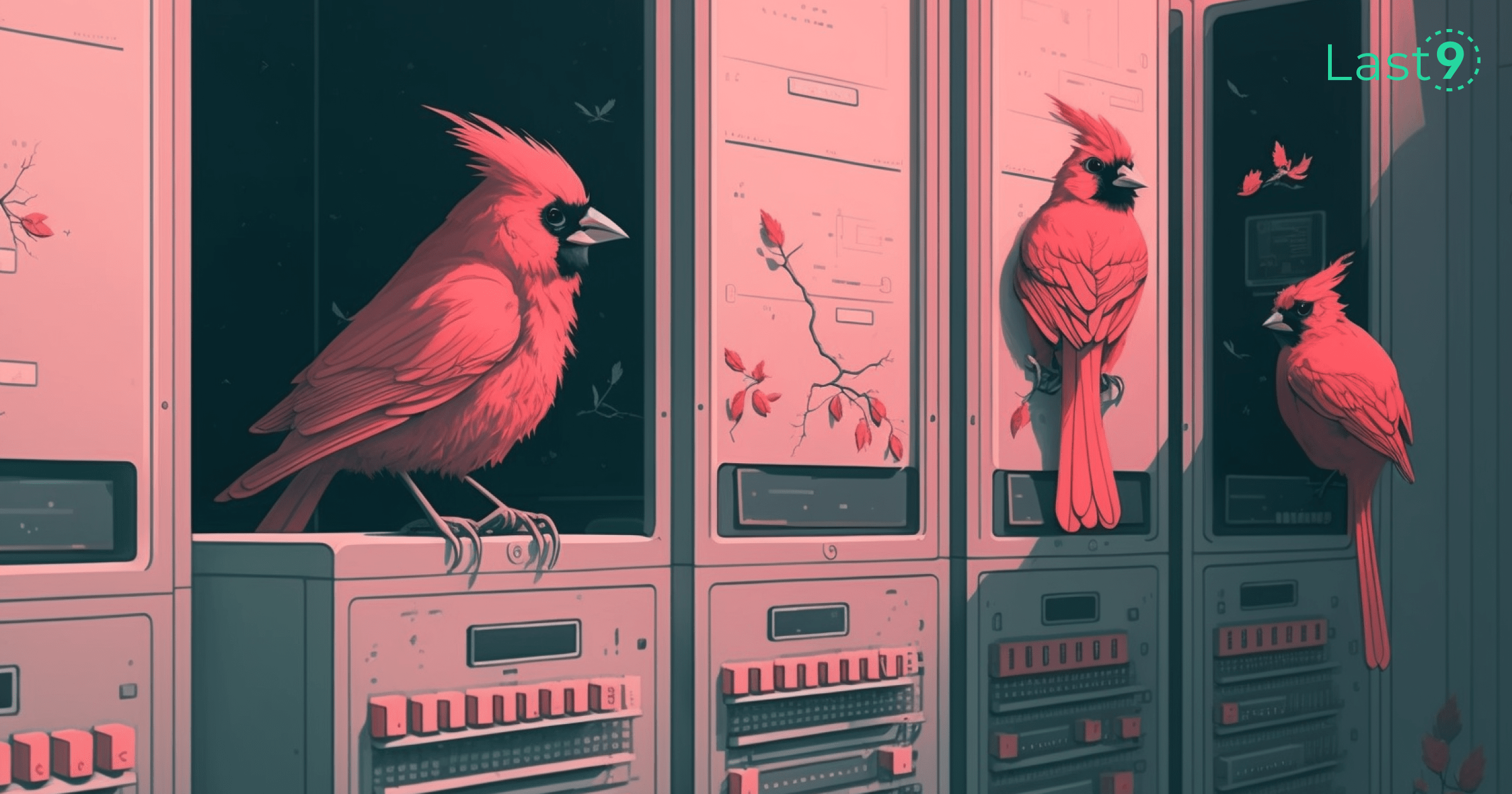
That brings me to my next question; how would you instrument if you didn’t have to bother about these restrictions? Would you do more with your metrics?