In software development, observability is key for engineers to get a real-time view of the inner workings of complex systems. Two popular tools that stand out are OpenTelemetry and Prometheus.
Both are CNCF graduate projects, but they serve different purposes when it comes to monitoring and debugging applications.
This article digs into what each tool offers, their pros and cons, and how they differ. Understanding their strengths and best-use cases will help developers choose the one that fits their needs best.
What is OpenTelemetry (OTel)?
OpenTelemetry (OTel) is an open-source framework for instrumenting, collecting, and exporting telemetry data from software applications. It helps provide insights into application performance and health using a collection of specifications, SDKs, and libraries.
Key features of OpenTelemetry:
- Vendor-agnostic: Offers libraries for different programming languages and frameworks.
- Flexible integration: Telemetry data can be exported to various backends, like Jaeger, Grafana, Prometheus, DataDog, NewRelic, Last9, and more, without altering telemetry processors.
- Unified observability: Formed from the merger of OpenCensus (data collection) and OpenTracing (distributed tracing), aiming to standardize observability across systems and languages.
OpenTelemetry is gaining popularity as the future of observability, with growing support from organizations and vendors alike.
How is OTel Built?
OpenTelemetry is designed with a modular, extensible architecture and standardized tools. Here are some of its key components:
- APIs:
OpenTelemetry provides language-specific APIs for popular languages like Java, JavaScript, Python, and Go. These APIs define the methods and interfaces that developers use to instrument applications and generate telemetry data. - SDKs:
Software Development Kits (SDKs) are libraries built on top of the OpenTelemetry APIs. They automatically capture telemetry data generated by instrumented applications and implement OTel APIs. The OpenTelemetry Collector then processes, filters, and exports this data in various formats. - Instrumentation Libraries:
OTel offers a vendor-agnostic model for instrumenting applications. It provides libraries that instrument popular frameworks and libraries (e.g., Spring, Express.js). This makes it easier to add telemetry to applications built on different languages and frameworks.
How Does OTel Work?
OpenTelemetry (OTel) helps you add application instrumentation through its APIs and SDKs, automatically directing system components to gather, analyze, and export telemetry data like logs, traces, and metrics.
With OTel, developers can easily add these data points to their code, simplifying data processing and export. Telemetry data is processed to filter errors and is then ready to be exported to a specified backend, such as an endpoint or a cloud-native computing foundation (CNCF) system.

Features of OTel
Automatic Instrumentation
OTel enables developers to initialize metrics, logs, and traces without modifying the application source code. This makes it easier to collect telemetry data for analysis.
Distributed Tracing
OTel allows you to trace transactions across various services within a distributed system, providing a clear view of request flows from the front-end to the back-end. This feature is essential for error detection and resolution.
Metrics Collection
OpenTelemetry supports the collection and analysis of metrics from instrumented applications. It focuses on deltas instead of cumulative values and supports integer metric values. It also allows extra metadata to be attached to histograms, enabling tracking of maximum and minimum values.
Additional Features:
OTLP (OpenTelemetry Protocol)
OTel uses OTLP for transporting telemetry data, providing a consistent, flexible protocol for data export.
Pipelines
OTel supports creating pipelines that help manage the flow of telemetry data, ensuring it is processed and exported to the right backends.
Prometheus Metrics
OpenTelemetry supports integration with Prometheus metrics, allowing data to be exported in a format compatible with Prometheus monitoring systems.
Client Libraries
OTel provides client libraries for different programming languages and frameworks, making it easy to instrument a wide range of applications.
Integration with AWS and Docker
OTel works well with AWS and Docker environments, making it suitable for cloud-native applications and containerized systems.
Why is OpenTelemetry Important?
OpenTelemetry plays a key role in modern observability practices by offering a standardized method for collecting and exporting telemetry data. Its ability to correlate metrics and traces makes troubleshooting and performance analysis more straightforward.
Advantages of OpenTelemetry
- Standardized and Easy-to-Adopt:
OpenTelemetry's approach to telemetry data collection is streamlined and easy to integrate, enhancing overall software observability. - Platform Integration:
OTel integrates well with a range of platforms and observability tools, including Prometheus, making it a versatile choice for developers. - Customization and Extensibility:
OpenTelemetry supports the development of custom exporters, plugins, and instrumentation libraries. Its automated instrumentation libraries help save time and effort by simplifying application instrumentation. - Comprehensive Telemetry Data:
OTel captures a wide range of telemetry data, including traces, metrics, and logs, providing a holistic view of application performance. - Wide Language Support:
OpenTelemetry supports multiple programming languages like Java, Python, JavaScript, and Go, making it adaptable for various tech stacks. - Active Community and Support:
OpenTelemetry is backed by a robust and active community, which includes industry experts. This ensures continuous development and integration of the latest advancements in observability.
Disadvantages of OpenTelemetry
- Complexity in Advanced Features:
OpenTelemetry's advanced features, like context propagation, distributed tracing, and custom exporters, can make it a bit tricky to handle. Since it involves embedding instrumentation code directly into the application being monitored, it can sometimes conflict with the principle of separation of concerns. This might require extra learning and expertise to manage effectively. - Ongoing Maintenance:
As an open-source tool, OpenTelemetry often requires continuous updates and maintenance as new versions are released. Keeping up with these changes can be time-consuming and may require additional attention. - System Resource Consumption:
OpenTelemetry's data collection and transmission processes can consume significant system resources such as CPU, memory, and network bandwidth. This could lead to increased overhead, requiring more resources and potentially impacting application performance.
What is Prometheus?
Prometheus is an open-source monitoring and alerting toolkit developed at SoundCloud designed to collect, process, and visualize metrics from various applications, using a flexible query language called PromQL to gain insights into application health and performance.
How is Prometheus Built?
Prometheus is built as a standalone platform. Here are some critical components of the Prometheus architecture.
Programming Language
Prometheus is primarily written in the Go programming language (Golang), which balances performance and development productivity well.
Time-Series Database
Prometheus uses a custom-built time-series database (TSDB) to store and query collected metrics. The TSDB is optimized for fast and efficient time-series data ingestion, storage, and retrieval.
Pull-Based Data Model
Prometheus adopts a pull-based model, periodically scraping metrics from instrumented targets. It supports various scraping protocols, including HTTP, HTTPS, and DNS.
PromQL
Prometheus’ flexible query language, PromQL, supports various functions, such as creating custom aggregation functions, for manipulating and querying time-series data. PromQL also facilitates complex queries, such as filtering metrics by labels and performing mathematical operations.
Monitoring and Alerting
Prometheus utilizes a web-based graphical visualization dashboard called the Prometheus Expression Browser for monitoring and troubleshooting. It also provides built-in alerting capabilities, allowing users to define alert rules based on specific thresholds. Prometheus can send alerts via various notification channels, such as email or Slack.
Exporters
Prometheus supports a rich ecosystem of exporters that collect application-specific metrics and export them in a format that Prometheus can scrape.
How Does Prometheus Work?
Prometheus works by scraping metrics from various targets, such as application servers, databases, or exporters.
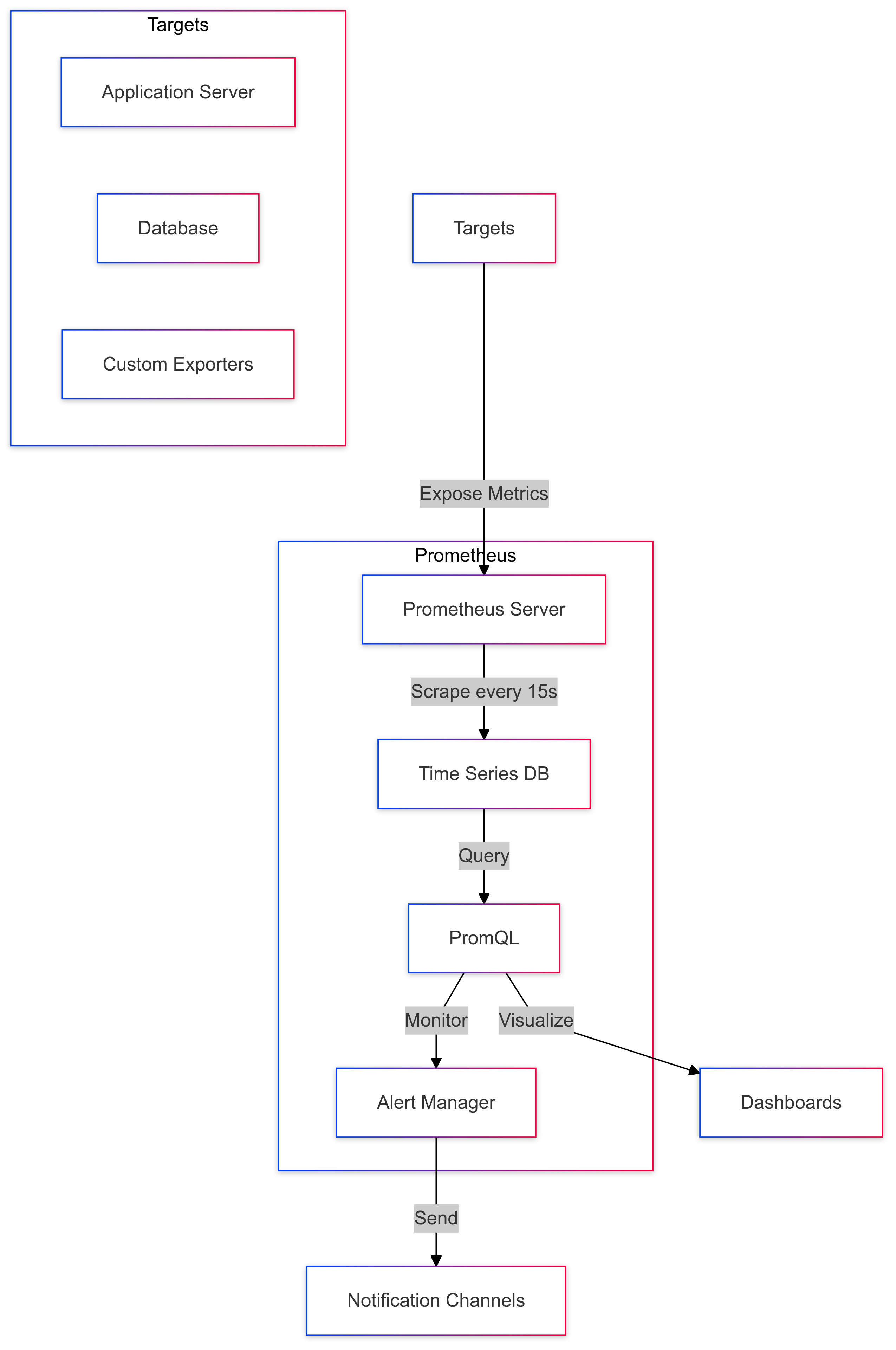
These targets are configured to provide relevant metrics, and Prometheus collects them at regular intervals. By default, Prometheus scrapes metrics every 15 seconds, although this can be adjusted.
Here's how it works:
- Scraping Metrics:
Prometheus pulls metric data from the configured targets, which could include system-level, application-specific, or custom metrics like CPU usage, memory usage, or request latency. - Storing Metrics:
The collected metrics are stored in Prometheus' time-series database. Metrics are organized by unique names, labels, and timestamps, making them easy to query and analyze. - Querying with PromQL:
You can define complex queries using PromQL (Prometheus Query Language) to analyze the collected data. PromQL supports functions, aggregations, and filtering to retrieve the specific metrics you need. - Alerts and Notifications:
Based on predefined thresholds, Prometheus can send alerts to your notification channels whenever certain conditions are met, helping you monitor and respond to system events effectively.
Features of Prometheus
Prometheus offers the following features.
Service Discovery and Target Management
Prometheus offers service discovery mechanisms to automatically discover and monitor new instances of services as they come online. It can integrate with service discovery systems like Kubernetes, Consul, and EC2.
Robust Querying
With PromQL, you can retrieve and analyze metrics, including functions, aggregations, and operators, using a flexible syntax. PromQL supports a range of operations for manipulating and querying time-series data, allowing software developers to create custom dashboards and alerts.
Alerting and Notification
Prometheus has a built-in alerting system that allows you to define alert rules based on specific conditions or thresholds. Prometheus generates and sends alerts via various notification channels when an alert condition is met.
Data Visualization
The Prometheus Expression Browser allows users to visualize metrics, create graphs, and explore data. The interface provides interactive features for zooming, panning, and applying various graphical options.
Hierarchical Federation
Prometheus servers are generally capable of monitoring large numbers of software components. But to make observability more cost-effective, Prometheus offers a hierarchical federation feature that allows software developers to configure a single high-level Prometheus server to collect metrics from multiple low-level servers.
Integration
Prometheus integrates with various tools and systems, such as alert managers, visualization platforms, and time-series databases.
Why is Prometheus Important?
Prometheus is crucial for software developers to monitor application events in real time. Embedding it during development helps you understand how different data types and systems interact, without needing to manually examine each architecture.
The ultimate goal of monitoring is to reduce errors, improve latency, and grow the user base. Users expect fast, reliable applications, and when performance falters, they may switch to alternatives, causing revenue losses.
Prometheus helps by tracking critical backend metrics like error rates and latency, allowing teams to quickly address issues and improve performance.
Beyond general observability, Prometheus also offers two key features: short-term storage and a visualization layer—advantages that OpenTelemetry doesn’t provide.
Advantages of Prometheus
Six key benefits of Prometheus are itemized below.
1. Prometheus is easy to set up and configure, requiring minimal overhead.
2. PromQL allows developers to perform complex queries, aggregations, and calculations on collected metric data.
3. Prometheus' built-in alerting system allows you to define alert rules and receive notifications when the set rules and thresholds are met.
4. Prometheus’ federation allows for easy horizontal scalability as monitoring needs grow.
5. It has a vibrant ecosystem with many exporters and integrations.
6. Prometheus allows for flexible and efficient storage, retrieval, and analysis of time-series data.
Disadvantages of Prometheus
Below are some critical drawbacks of Prometheus.
1. Prometheus relies on scraping metrics data from targets, which may introduce long-term delays and scalability challenges, especially in heavily loaded or distributed environments.
2. Prometheus focuses primarily on metrics collection and does not provide native support for distributed tracing.
3. While Prometheus is built to handle large-scale deployments, it may face challenges with long-term data storage. Though it retains data for a configurable retention period, users need to consider external solutions for historical data storage, making it a resource-intensive monitoring solution.
4. Prometheus focuses primarily on metrics-based monitoring, so users have to select other tools for traces and logs.
OTel vs. Prometheus Quick Comparison
The table below presents some crucial differences between OTel and Prometheus.
Feature | OpenTelemetry (OTel) | Prometheus |
---|---|---|
Purpose | Unified framework for collecting, processing, and exporting telemetry data (metrics, traces, logs). | Specialized tool for collecting and storing time-series metrics. |
Data Types | Supports metrics, traces, and logs for comprehensive observability. | Primarily focuses on metrics collection and querying. |
Data Collection | Uses automatic instrumentation, SDKs, and APIs for data collection across multiple layers. | Scrapes metrics from predefined targets at regular intervals. |
Integration & Flexibility | Vendor-agnostic, integrates with various backends (including Prometheus). | Focused on integration with Prometheus exporters and other related tools. |
Querying | Data exported to various backends for querying, supports flexibility. | Uses PromQL for querying time-series metrics. |
Storage | Relies on external backends for data storage. | Has a built-in time-series database for metric storage. |
Alerting | Alerting capabilities depend on the backend system. | Built-in alerting system with flexible thresholds and notifications. |
Visualization | Supports integration with tools like Grafana for visualization. | Built-in visualization layer with native integration to Grafana. |
Use Case | Best for comprehensive observability with support for multiple telemetry types. | Best for monitoring and alerting based on time-series metrics. |
Metrics in OpenTelemetry vs. Prometheus
The semantic convention for metrics in OpenTelemetrythe (OTLP metrics) does not align with Prometheus' native metrics naming convention. This means that metrics in OpenTelemetry and Prometheus do not have the same format and specification.
Check out following video to understand differences between OpenTelemetry Metrics and Prometheus
To address this disparity, there is a module in otel-collector-contrib
that offers centralized functions that facilitate the conversion of OpenTelemetry metrics into metrics compliant with Prometheus.
Metrics Comparison: Prometheus vs. OpenTelemetry
Aspect | OpenTelemetry (OTel) | Prometheus |
---|---|---|
Data Format | Supports various formats: - OTLP (OpenTelemetry Protocol) - Prometheus exposition format - Other vendor-specific formats | Uses the Prometheus exposition format (text-based) which is HTTP-compatible. |
Metric Schema | - Uses metric types (counter, gauge, histogram) - Labels can be dynamic and attached with extra metadata | - Fixed schema with metric names, labels (key-value pairs), and metric types (counter, gauge, histogram). |
Naming Convention | Metric names follow the format: <metric_name>_<metric_type>_<suffix> . Example: http_requests_total | Simple format: metric_name (e.g., http_requests_total ). Names are lowercase with underscores. |
Labels/Tags | Flexible labels or tags can be used for extra context (e.g., service names, request types). Labels can vary by instrumented component. | Uses labels (key-value pairs) for context (e.g., method="GET" , status="200" ). Labels are often more fixed and predefined. |
Metric Types | Supports counters, gauges, histograms, and summaries. More flexibility in defining data points. | Supports counters, gauges, and histograms with less flexibility in how they’re defined. |
Units | No strict unit convention, but SI units are recommended (e.g., seconds for latency, bytes for memory). | Typically uses unit-less counters or seconds (e.g., duration_seconds ). Units are implied based on metric name. |
Summary
Choosing the proper observability framework for your specific application and infrastructure needs is critical.
While OpenTelemetry and Prometheus are potent tools, OpenTelemetry provides a more comprehensive approach to observability across multiple platforms and languages, supporting metrics and distributed tracing.
An in-depth comprehension of the highlighted capabilities and differences will allow you to make informed decisions and use the proper framework for your observability needs.